In today’s healthcare landscape, diagnostic accuracy is both more critical and more difficult than ever. With the exponential growth of medical data, complex symptom patterns, and an overburdened system, physicians face mounting challenges in catching diseases early and accurately.
Artificial Intelligence (AI) is not a replacement for clinical expertise—it’s a powerful ally. With its ability to process thousands of data points in seconds, AI is opening a new chapter in diagnostic medicine. This article explores how AI helps uncover hidden patterns in medical data, speeds up disease detection, and improves patient outcomes—while addressing the ethical and technical considerations involved.
The Diagnostic Dilemma in Modern Medicine
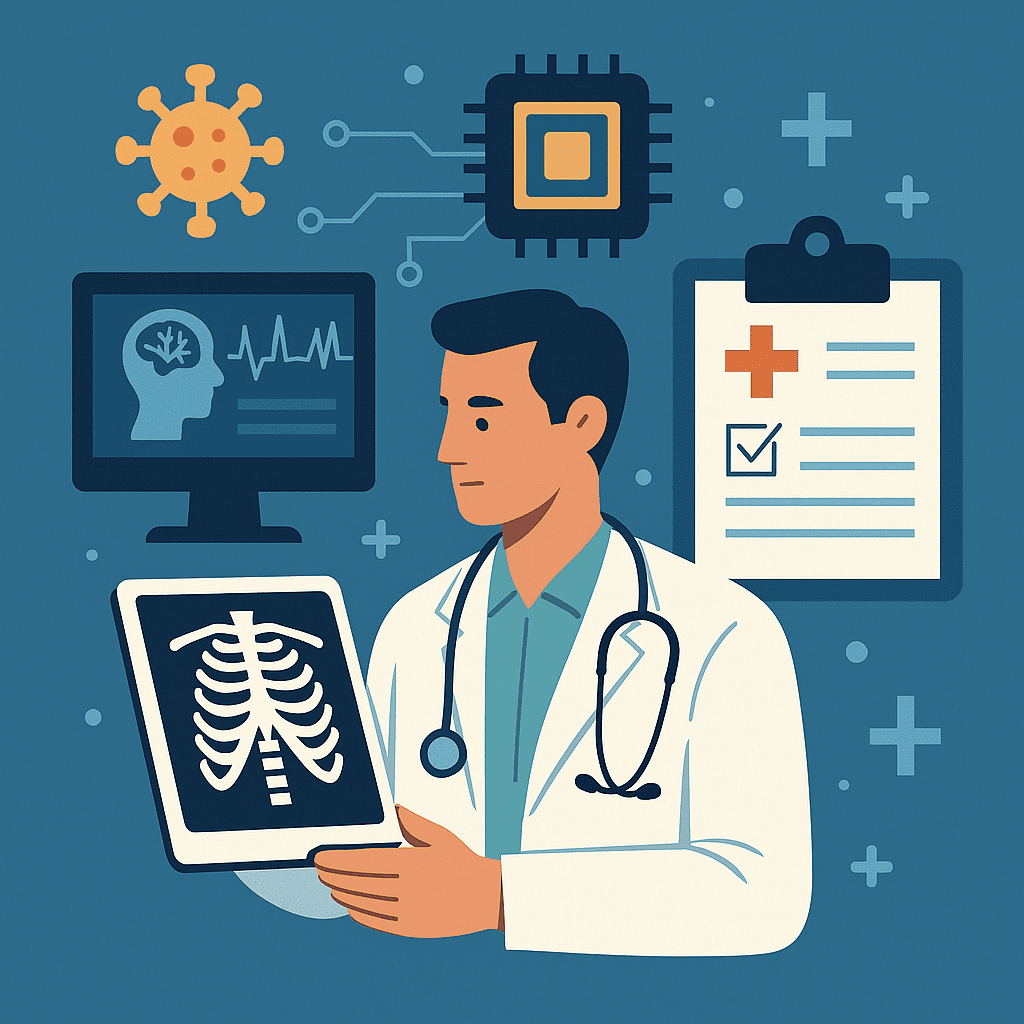
Medical professionals today must interpret enormous volumes of data, from lab results and genetic profiles to years of patient history. Symptoms often overlap between common and rare diseases, and even experienced clinicians can miss early indicators hidden in a patient’s full health timeline.
Reports show that diagnostic errors are responsible for roughly 25% of all medical error-related deaths in the U.S.—a staggering 64,000 preventable deaths annually.
AI helps mitigate these risks by supporting healthcare professionals with advanced analytical capabilities that go far beyond human limits.
What AI Brings to the Table
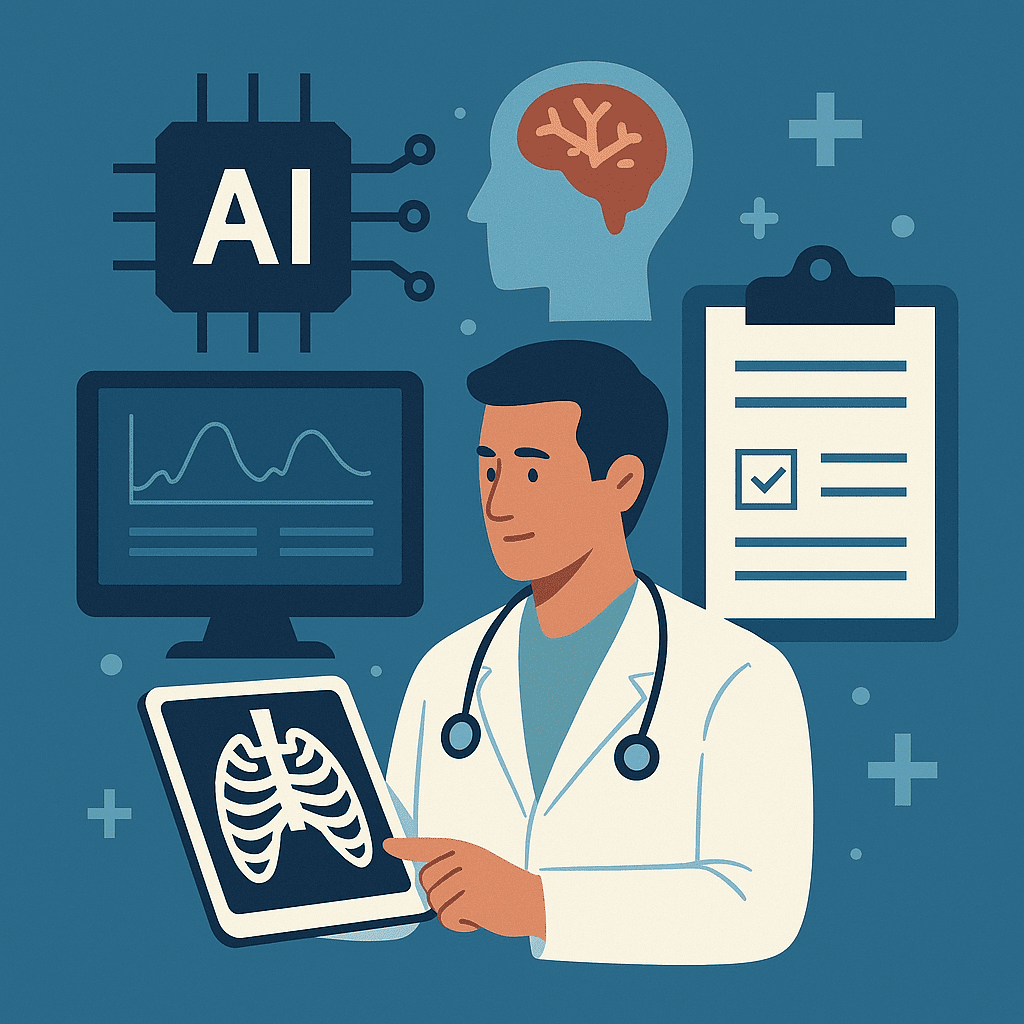
AI-powered systems are already proving their value:
- 🔍 Pattern recognition across massive datasets, flagging early disease signals
- 📊 Analysis of unstructured data like doctor notes and medical images
- 🧬 Prediction of disease risks based on genetic markers and long-term trends
- ⚖️ Balancing rare vs common diagnoses, without bias or fatigue
One powerful example is Harvard’s CHIEF system (Clinical Histopathology Imaging Evaluation Foundation). Trained on thousands of cancer images, CHIEF detects 11 different types of cancer with 94% accuracy—even identifying key genetic mutations that influence treatment decisions.
Real-World Use Case: AI Processes 15,000+ Data Points
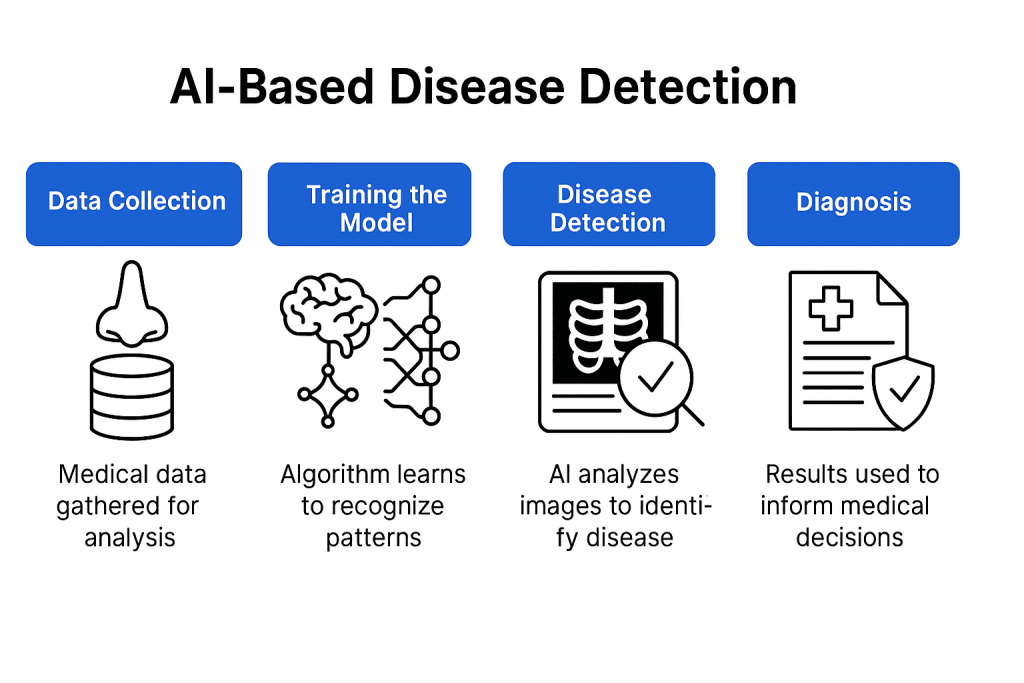
Imagine a patient with symptoms like fatigue and joint pain. Her EHR might contain:
- 15 years of lab results
- 8 specialist consultations
- 6 imaging scans
- 2 genetic profiles
- 47 medication records
Traditional diagnosis might focus on recent data—but AI can analyze the full spectrum, connecting subtle clues that only emerge when viewed across years and data types. This approach helps surface diagnoses earlier and with higher confidence.
Case in Point: UCLA’s SLIViT – Self-Learning AI for 3D Medical Imaging
Developed at UCLA, SLIViT (Slice Integration by Vision Transformer) is an AI model that interprets complex 3D scans—like MRIs or CTs—with near-expert accuracy. What’s remarkable is its ability to train on hundreds, not thousands, of scans. This dramatically reduces the time and cost of building reliable diagnostic tools.
SLIViT has been applied to:
- Retinal scans to detect early-stage eye diseases
- Echocardiograms for heart function analysis
- Liver MRIs for staging fibrosis
- Chest CTs for cancer screening
These systems not only match specialist performance—they do so with a fraction of the resources and time.
Ethical and Technical Considerations
Despite the promise, implementing AI in healthcare comes with real challenges:
- 🔐 Data privacy: Medical data must be stored and processed securely
- ⚖️ Bias mitigation: AI models must be trained on diverse data to ensure fair outcomes
- 🧩 System integration: AI must complement, not disrupt, existing clinical workflows
- 📣 Transparency: Clinicians must understand and trust AI-generated insights
At Tino Agency, we work closely with medical institutions and startups to ensure AI tools are deployed responsibly—with careful attention to data governance, regulatory compliance, and usability.
Why This Matters for Healthcare Organizations
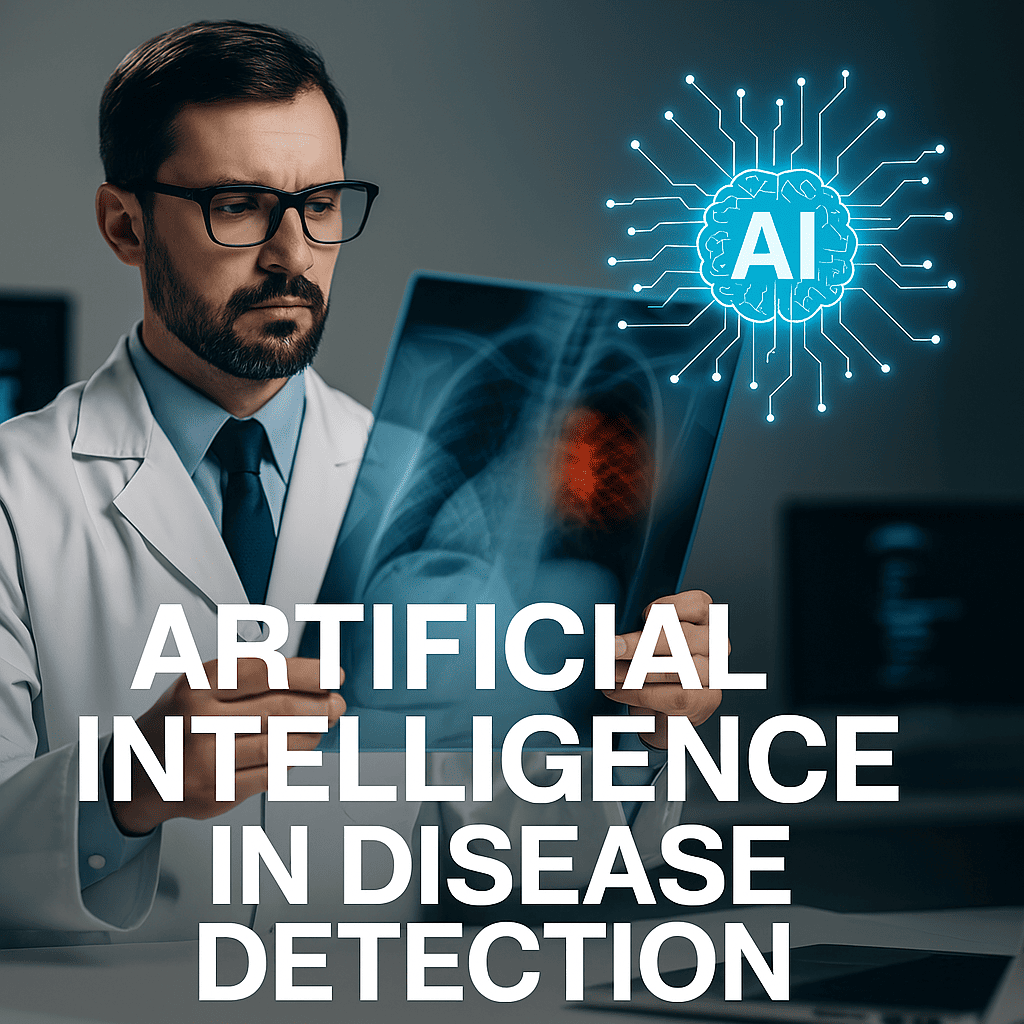
By integrating AI into diagnostic systems, healthcare providers can:
- Reduce misdiagnosis and delays
- Detect diseases months earlier
- Free up clinicians to focus on patient care
- Create scalable, data-driven workflows
Whether you’re building a medical imaging platform, a diagnostic support tool, or a clinical decision engine, AI isn’t the future of healthcare—it’s the present.
FAQ
AI analyzes complex medical data—including notes, images, and labs—to detect patterns that may not be visible to the human eye.
No. AI supports clinical decisions but does not replace the expertise and human judgment of trained physicians.
Ensuring data security, avoiding algorithm bias, and making AI insights understandable and actionable for clinicians.
Absolutely. At Tino Agency, we help healthcare startups and providers design, develop, and integrate AI into medical products—from prototype to production
📞 Let’s talk about your idea. Schedule a free consultation to explore what’s possible with AI in your healthcare product.